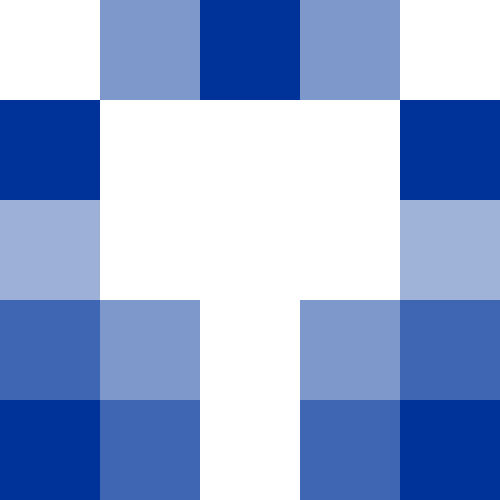
Developers & AI
Episode Ten
Intro
I'm Peter and this is the RoguePlanetoid Podcast where you will find insights about Microsoft or related platforms and technology, along with so much more whether you are beginner or an experienced professional or just interested in technology. Keep Current, Keep Coding!
Welcome
Welcome to episode ten of the RoguePlanetoid Podcast about Developers and AI but first I'd like to thank Jamie Taylor for having me as a guest on The Modern .NET Show in the episode Unleashing the Power of Windows Development. It was great to talk with Jamie about my journey as a developer creating experiences for Windows and the Windows App SDK! It was also great to see so many new listeners discover the RoguePlanetoid Podcast thanks to that appearance. I really appreciate everyone who has taken the time to listen to this podcast. You can listen to my episode of the Modern .NET Show by searching for Unleashing the Power of Windows Development with Peter Bull wherever you listen to your podcasts, or you can check out the link in the show notes.
Overview
RoguePlanetoid Podcast started just a few weeks after the emergence of the first major Large Language Model, ChatGPT which helped usher in the next evolution of generative AI. In the months since then, there has been unprecedented growth and adoption of this new technology and has become the fastest growing new technology of all time even faster than other technologies such as social media. AI shaped by developers will help deliver the Microsoft vision to empower every person and every organization on the planet to achieve more. Microsoft Build AI Day was recently held in London talking about generative AI having the potential to reach every part of society in every part of the world, and it is the role of developers to produce the experiences and applications of this new technology.
OpenAI
OpenAI revolutionised generative AI with ChatGPT that allowed anyone to type what they wanted and get what they needed, this was a game changer in the world of user interface design where natural language became the way anyone could interact with ChatGPT to learn, have fun, or just create content. ChatGPT allows you to get answers to questions, create original content along with remembering what was said in a conversation allowing for clarifications and corrections. Not only did ChatGPT make it possible to create amazing text-based content, but it was also possible to create image-based content with DALL-E which could create images based on descriptions. DALL-E allows you to create realistic looking images combining concepts, attributes along with styles just by describing what you want with a text description. Generative AI to create either text or images made it possible for anyone, not just developers or experts to use these tools and as the technology has advanced, it has become even more capable with later versions of the GPT and DALL-E models becoming more even sophisticated without becoming more complex to use, a common trope usually expected with advances in technology.
However, OpenAI are not alone in the spaces in large language models and generative AI, but they are at the pinnacle of that domain with alternatives from Google, Meta and others being created but OpenAI still dominates the field of generative AI. OpenAI provides opportunities for developers with their API to take advantage of GPT-3 or GPT-4 in their applications or to create plugins that enhance those experiences to provide additional functionality. This ability to create plugins means that developers can extend their own experiences into the generative AI space by providing context and functionality from their services into ChatGPT. If you want to find out more about OpenAI as well as try out ChatGPT and DALL-E for yourself then visit openai.com or check out the link in the show notes.
Next generation AI for developers & Azure OpenAI Services
Scott Hanselman spoke at Microsoft Build AI Day in London talking about next generation AI for developers and about large language models potentially crossing the line into creepy territory, if the context of something that a large language model means it produces something about yourself you didn't expect given a certain context, then it could potentially come across this way based upon what a large language model may have access to. The more context you give a large language model the better the responses can be but there may be a point where those responses sound too invasive, but it is possible to guide a large language model such as making it pleasant as a helpful assistant, but it is also possible to make a more belligerent assistant that isn't happy about answering questions and is more reluctant to do so. Scott went on to say that a large language model is essentially a sock puppet - if you tell it to take over the world then the hand that makes it do that is your own, if you tell it and train it to do horrible things, it will do horrible things. Making large language models work for everyone is going to need responsibility and thoughtfulness from developers. Large language models can become more random by changing temperature, the closer to zero the more repetitive and deterministic the large language model will become but turn up the temperature too high and it will become too chaotic and with Azure OpenAI it is not possible to turn this up too high. This change in temperature can be abstracted from users such with Bing Chat where More Creative represents a higher temperature and More Precise represents a lower temperature, but these parameters are delicate so developers will need to experiment and perform testing to make sure they are getting the outcomes they want from their large language models.
Scott Hanselman also said during Microsoft Build AI Day that developers creating enterprise experiences will want to ground their models with the context of what is needed from the application and make sure it doesn't go off and do other things. Grounding is used to inform a large language model of what it is meant to be good at and needs to know about what people are asking, much of this experience has already been done with the development of search engines where you provide what you need to get the outcomes you want. How do you prevent a large language model from coming up with problematic answers, this can be mitigated by layers of safety including at the model layer and this mitigation is based on a lot of understanding from both OpenAI and Microsoft, which includes identifying unsuitable content being input to or output from a large language model. Developers can also consider adding rules into the system message which can be used to guide a large language model or provide context such as refusing to engage in any argumentative behaviour. However, people can try to inject things into the model and get it to do things, so rules can be used to help prevent this jailbreaking of the model and this should include not allowing the rules themselves to be disclosed by the model, preventing the issue where people can ask for the rules and then have the model disobey those rules. Developers can catch issues higher up in an application as well as the OpenAI Service catching things going in and going out. Since large language models essentially have one large text area as the user interface then you need to not only respect how information will be presented to the user but also, as with creating websites you can't trust anything input especially as that large text area allows people to paste anything they want and could make your model do things it wasn't designed to do.
Henk Boelman also spoke at Microsoft Build AI day about getting started with generative AI using the Azure OpenAI service and mentioned that the future is AI using models that are flexible and can be applied to any task, such as foundation models trained on a lot of unlabelled data, which can be very complex and require a lot of resources such as GPT-3 which has 175 billion parameters and took millions of dollars and many days to train the model on Azure infrastructure. Azure Open AI service is a platform that offers enterprise security for your data and keeps it private and doesn't use it to train other systems or models. Large language models take in several tokens then produce one token out, although this token is from a list of probabilities where the most likely token is returned, and tokens are produced until a stopping condition has been reached. With the system prompt this is where you can program and steer your model, so can start with response grounding to make sure that it for example responds in a factual way and not add any additional information, along with tone where responses are polite, and for safety you can make it decline responses that may hurt people and if someone asks about the rules the model can state that they are confidential and decline any changes.
Henk also said during Microsoft Build AI Day that if you want to have structured data back from unstructured data then you can support the calling of functions where you can have data returned in the format that you need. You use the Azure OpenAI service on your data, but the models themselves were fixed at the point they were trained, so they are not retrained on your data and will not contain any of the knowledge from your company, so you need ground the model with your data so that the model can reason over this data, which is not learning. Developers control the access to this data which can use a vector database that can retrieve relevant data to construct the prompt which can integrate both internal and external data sources and be combined with both structured and unstructured data. You can find out more about the Azure OpenAI service at azure.microsoft.com/products/ai-services/openai-service or check out the link in the show notes.
GitHub Copilot
GitHub Copilot started two years ago as a comparatively early effort in the development of generative AI and large language models given the pace of change since it was released and was trained on the English language, public GitHub repositories along with other publicly available source code. OpenAI provided the technology behind GitHub Copilot which gave developers the ability to type a comment for the functionality they wanted and then get code suggestions based upon that comment. The main aim of GitHub Copilot was to reduce the amount of boilerplate code needed to be written by developers so they could focus on the problems they needed to solve and keep them in their flow, rather than reproduce or replicate already solved solutions.
Chris Reddington from GitHub spoke at Microsoft Build AI Day in London explaining that GitHub Copilot is about text prediction and is not a compiler, it is just predicting text and there is no understanding of the programming language, so you need to work alongside it, it is called Copilot for a reason - it is not an autopilot. GitHub Copilot has benefited from advances in generative AI and now features the more up to date and sophisticated models but also introduces new features such as GitHub Copilot Chat where developers can ask for the functionality they need or refine requests to get what they need. When you use GitHub Copilot on existing projects it will have the context of any existing patterns along with being able to see the way you do things, and it will be able to assist you more compared to a blank project.
Chris also said at Microsoft Build AI day that GitHub Copilot can't read your mind, it is all about communicating and being specific about your intent, if you expect short answers back then you are getting what you need back, but if not, you can iteratively go over what you need to get what you want. With GitHub Copilot you may need to go back and forth incrementally especially if you don't get the response from your prompt that you expected, it is all about getting the direction you need and you can add more or rewrite your prompt, also you can get context from classes that can be included to bring it all together.
With GitHub Copilot you don't need to blindly accept what you get, although you can if you want to and with new features such as GitHub Copilot Chat which can be used for rubber ducking scenarios as a developer you can bring any suggestions into the codebase. These suggestions still need to be reviewed just like with any other code and there is still need for developers to solve specific problems and get the most out of GitHub Copilot, developers can also share the ways they have got things from it to help others get what they want from it. GitHub Copilot has helped thousands of developers throughout the world increase their productivity with features such as the ability to create Unit Tests, or even ask if there are any security vulnerabilities in the code and get the solutions to resolve them. It is fitting that developers were the first to benefit from the field of generative AI and now developers are helping to push the boundaries of what is possible with generative AI. Find out more about GitHub Copilot by visiting copilot.github.com or check out the link in the show notes.
What really is Generative AI?
Seth Juarez also spoke at Microsoft Build AI Day in London and helped explain exactly what generative AI is, and the type of impact it has had that's been very different to previous advances in technology. Seth said that usually it is people in the technology industry telling everyone else to use something but with everyone else says they're not going to do it. However, with generative AI it has been everyone else saying generative AI is important and those in the technology industry were caught a little off guard by this. Large Language Models take in a fixed number of tokens which are made up of pieces of words, so rather than having every type of text these are broken up into pieces of around four characters and when you get a token output this is actually from an array the size of the dictionary, with the probability of the next word being the most likely and depending on the factor known as temperature will control which of those tokens are returned and so on to the next token until the output is complete. This is why a large language model looks like it is typing as the next token is selected but this can seem to give the model human-like qualities, but this is a misunderstanding. Never assume a large language model knows anything, all it is a language calculator, so the main job is to put the right stuff in so the likelihood of what you want comes out, as developers we need to validate and test inputs and outputs. Although AI may not be dangerous on its own, if you give those kinds of systems agency then it could go wrong.
Seth Juarez also said at Microsoft Build AI Day that when using Azure Data with large language models your data is being added to the prompt not the model and any inputs or outputs are not available to any other customers or any other AI. However, there is a content filter for both input and output that is built into the service which can help prevent abuse which can be opted out of, but should you do so, then if a model is abused then it is up to the developer to respond to that abuse rather than Microsoft. If developers want to add more data and have more control with a Large Language Model, then it is possible to use Prompt Flow which helps orchestrate flows into the prompt. This could be information about customers or documents along with data from a database and these Prompt Flows can be validated and tested and you can evaluate that any answers are grounded. Answers may be wrong but as long as someone can correct it then that is okay, as long as responses are factual and can check if answers are grounded in the context that was needed. Seth also said that developers can check how grounded a model is, by outputting the context and perform testing using a large language model adversarially against your model as a language calculator to score those outputs from one to five, to see what good or bad answers are being returned. Developers can deploy their models and then turn on the collection of data to store the actual input and output of the data and monitor when grounding of a model goes down. To read more about Microsoft Build AI Day with insights from Scott Hanselmann, Henk Boelman, Chris Reddington and Seth Juarez then you can read my article at rogueplanetoid.com/articles/microsoft-build-ai-day or check out the link in the show notes.
Overview
Generative AI is a truly transformative technology that is leading the way with unprecedented adoption across not only in the technology industry but in the general population too. Satya Nadella CEO of Microsoft spoke at Microsoft Envision in London and talked about multi modal, multi turn and multi domain generative AI is having the biggest impact for the user interface compared to the development of the PC or the smartphone. Satya went on to say that AI needs to be balanced with the risks and concerns about the usage of it, so we need to keep talking about the capabilities and impact along with any unintended consequences and not wait for it to potentially have a devastating impact on society. Satya also stated there will be a structural shift when it comes to jobs and skills where some will be automated and others will mean different skills, although these will be able to be acquired on the job so that the skills and talent come together. These new skills will act as a signal for other jobs that will be created and many of those will need different skills, and if that takes time then we can use Large Language Models themselves to acquire those skills, as they are a great leveller in terms of working your way up the skills ladder and jobs will appear with better wages and support to drive productivity within companies with skills to drive monetisation. Microsoft is leading the way with AI including accelerating productivity with AI features built directly into Microsoft tools and services, allowing developers to create their own AI-based tools and services all while ensuring that principles have been put into practice and AI functions as intended. To find out more about building the future faster with Microsoft AI then visit microsoft.com/ai or check out the link in the show notes.
Outro
Thanks for listening to the RoguePlanetoid Podcast where each episode you will find insights about Microsoft or related platforms and technology, along with so much more wherever you listen to your podcasts or at rogueplanetoid.com/podcasts for the RoguePlanetoid Podcast whether you are a beginner or an experienced professional or just interested in technology. Keep Current, Keep Coding!
- Website - rogueplanetoid.com/podcast
- X - x.com/rogueplanetoid
- YouTube - youtube.com/@rogueplanetoid
- Unleashing the Power of Windows Development with Peter Bull - Modern .NET Show - dotnetcore.show/season-6/from-silverlight-to-windows-app-sdk-unleashing-the-power-of-windows-development-with-peter-bull
- OpenAI - openai.com
- Azure OpenAI Service - azure.microsoft.com/products/ai-services/openai-service
- GitHub Copilot - copilot.github.com
- Microsoft Build AI Day - rogueplanetoid.com/articles/microsoft-build-ai-day
- Microsoft AI - microsoft.com/ai
RoguePlanetoid Podcast is a production of cluarantonn.com
Hosted, Written, Produced and Edited by Peter Bull
Music based on Like a Tiger by Jo Wandrini
Production Company Name by Granny Robertson